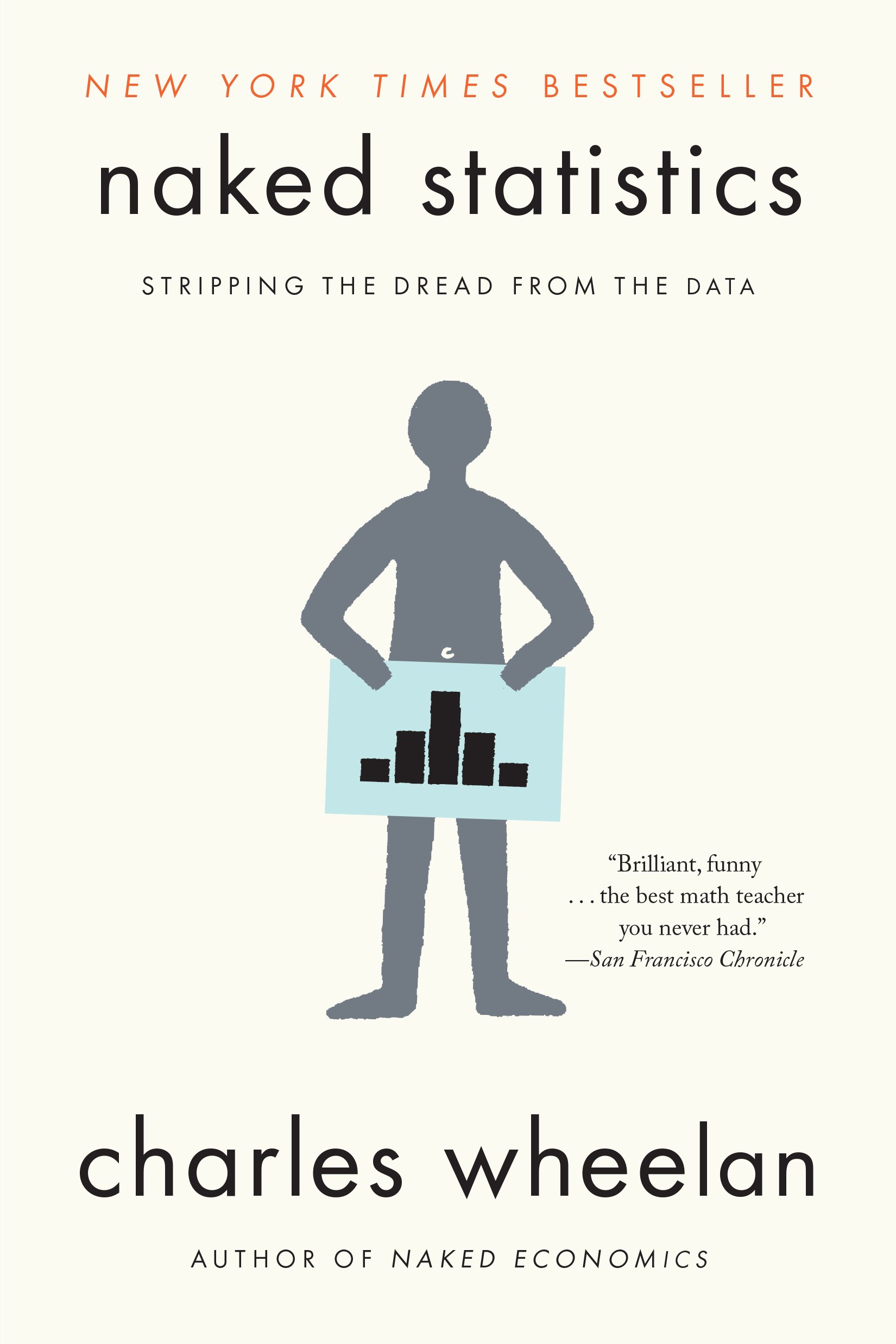
Summary and Review: Naked Statistics
Stripping the Dread from the Data
✍️ The Author
This book was written by Charles Wheelan and published in 2014. Wheelan is a lecturer at Dartmouth who teaches coureses on topics related to policy and economics.
💡 Thesis of the Book
Statistics is a profound tool for inferring truths from data, not proving them, and ultimately it has no say on what questions we ought to seek answers to. That judgement is reserved for the human. We decide how to employ statistics, and it can do much good when used properly and much harm when misused. Statistics can be used to lie, but it is crucial for telling the truth.
💭 My Thoughts
Wheelan explores some of the most profound concepts in the field of statistics through intuitive explanations, examples, and humor. It was a fun read, but even as a data scientist, it added value. I actually found myself grasping fundamental statistical concepts in a more intuitive manner, even though I have employed all these tools to solve data problems many times. I truly wish someone handed me this book before I took my first statistics class. Additionally, Wheelan’s writing has inspired me to strive towards his level of explanatory capability.
📕 Chapter Summaries
Introduction: Why I hated calculus but love statistics
We are often taught mathematical topics without context of their application. An apparent lack of purpose can make these topics seem pointless, and humans tend to find what is pointless to be boring. This is precisely why Wheelan hated calculus but loved statistics, because the former was fed to him in the form of dry equations and the latter was fed to him in the form of fascinating real-world context. If you can understand the intuition behind statistical concepts, the mathematics becomes a lot more clear, so the purpose of this book is to explain the intuition of the most basic but profound statistical concepts through the use of story, humor, and logic.
Chapter 1: What’s the Point
Statistics can help us answer questions, understand large datasets, and learn about the world through the analysis of our measurements. Given a large dataset with many rows and columns, we can’t simply make sense of it by looking at it, so we uses statistical techniques to simplify the data so that we can describe it, find relationships, and make comparisons. This process results in plenty of information reduction, but nevertheless it is the only way data can inform us. While statistics can help inform us, it is by no means a way of representing absolute truth. It goes hand-in-hand with human judgement to make sense of the data. The questions we ask, the aggregations we perform, what we should measure, how we should collect data, and our interpretations of the numbers are left to human judgement, not statistics. Statistics can be wielded to mislead just as easily as it can be wielded to inform, depending on the human that wields it.
Chapter 2: Descriptive Statistics
Humans cannot intuitively make sense of large volumes of data without performing some kind of aggregation as a way to simplify many numbers into few numbers. This forms the basis of descriptive statistics. Its strength is also its weakness, as information reduction also means information loss, which is why we should avoid mistaking descriptive statistics as merely truth. It is a simplistic representation, not a true one, but that simplification makes it possible for us to make sense of data. Two profound descriptive measures are “central tendency” and “dispersion”. Mean and median are measures of central tendency, while standard deviation and variance are measures of dispersion. The choice of measure crucially depends on the context, as making the wrong choice can lead to incredibly erroneous conclusions. This is why rankings are a tricky thing, because they rely on a series of aggregations that are weighted based on perceived importance. Selecting the wrong aggregation type can produce misleading rankings, not to mention the subjective element of weighting the various aggregations (who decides what is important?). When presented with descriptive statistics, always carry a bit of caution and ask for multiple representations of the measure.
Chapter 3: Deceptive Description
Statistics can lead us in the right direction, but it can also lead us in the wrong direction. Precision does not imply accuracy. Numbers are not truth. Statistics can be deceptive, and striving to improve arbitrary metrics can produce unintended consequences. You can arrive at entirely different conclusions just by changing the unit of analysis, switching between mean and median, and looking at absolute change versus percentage change. Often when we set a metric to improve, it incentivizes the wrong behaviors. There is an old saying, “what gets measured gets managed”, but be sure you are measuring what you want to manage.
Chapter 4: Correlation
Correlation is a profound tool that has been leveraged to identify relationships between variables. It describes a statistical pattern amongst two or more variables, where a change in one variable is associated with a change in another variable. While this tool is powerful for identifying relationships, it does not establish causal relationships. This is the underlying idea behind the old maxim “correlation does not imply causation”.
Chapter 5: Basic Probability
In any instance where we are dealing with uncertain phenomenon, probability is relevant. We don’t definitively know the outcome of an uncertain process, but we can estimate how likely each outcome is using probability theory. There are known probabilities, probabilities inferred from data, and probabilities computed by models. One of the most profound laws in probability theory is the law of large numbers, which states that as more trials are conducted, the frequencies of each outcome converge to their probabilities. This simple law explains why it is in your best interest to make good decisions, even though they don’t always yield the good outcomes you expect from them. As you make more decisions, the outcomes will converge to a net positive. This law also supports the utility of expected value analysis, where you make decisions based on the expected values (sum of outcomes weighted by their probability). When you gamble or buy insurance, the expected value is never in your favor because the business models of those industries rely heavily on the law of large numbers and a positive expected value.
Chapter 6: Problems with Probability
Probability and statistics provide us mathematical tools for dealing with uncertainty, but those tools are not any smarter than those who wield them. There are several common mistakes people make when employing probability: assuming events are independent when they are not; not understanding when events are independent; failure to recognize improbable things are likely to happen when many trials are conducted; succumbing to prosecutor’s fallacy; ignorance of the influence of mean reversion in variables subject to chance; using statistics as justification for discrimination.
Chapter 7: The Importance of Data
The utility of statistical methods is constrained by the quality of data you employ them on. The famous “garbage in, garbage out” maxim holds true. You cannot possibly achieve profound results if you run statistical analysis on bad data. Data collection processes make up their own subject of study because of how important they are to any downstream analysis. In science, it is often impossible to collect data on an entire population, so scientists have to sample that population. If the sampling process is biased, you get a sample that is not truly representative of the population, so any conclusions drawn from analyzing that sample will incorrectly be assigned to the population. The ideal sampling process is random, meaning every observation in the population has an equal chance of being selected.
Chapter 8: The Central Limit Theorem
One of the most profound statistical concepts is the central limit theorem. This theorem forms the foundation for much of statistical inference involving samples and populations. Assuming a proper sampling process and large enough sample sizes, CLT posits that the means of all samples drawn from a population will form a normal distribution around the population mean, regardless of the probability distribution of the population. This theorem lets us make 4 types of inferences: 1) we can infer about random samples based on what we know about the population; 2) we can infer about a population based on what we know from its random samples; 3) we can infer whether or not a sample belongs to a population based on what know about the sample and population; 4) we can infer whether or not two samples were drawn from the same population based on what we know about each sample.
Chapter 9: Inference
Inference is not about proving a hypothesis. It is about assessing the validity of a hypothesis based on the probability of observing the data if it were true. This is the underlying idea behind statistical hypothesis testing. If it is very unlikely we would make some set of observations in a universe where the null hypothesis is true, then we can reject that hypothesis with some degree of confidence. Conversely, if it is quite likely we would make some set of observations in a universe where the null hypothesis is true, then we can accept the null hypothesis with some degree of confidence. Scientists frame an experiment in this manner, where they have some hypothesis about a relationship or effect, and they test its logical complement (there is no relationship or effect). If you can reject the null hypothesis, then the data is said to be in support of the scientist’s hypothesis (called the alternative hypothesis). There is a crucial distinction between inferring a hypothesis is true and proving a hypothesis is true, and science is grounded in the former, not the latter.
Chapter 10: Polling
Polling is a classic example of the central limit theorem because you are trying to infer the proportion of a population that holds some belief based on the responses of a representative sample. If the sampling process suffers from selection bias, then the inference no longer works. Polls are especially susceptible to self-selection bias, where the segment of people that respond to the poll may be fundamentally different from the segment that does not respond. Even in an unbiased sample, there is still room for error. That is why polls are reported with a “margin of error”, which is just the 95% confidence interval. It is easy to infer the opinions of a population based on a properly drawn sample. The tricky part is drawing a representative sample that gives accurate responses to the questions asked.
Chapter 11: Regression Analysis
Regression analysis is useful for isolating the association between two variables when there are multiple explanatory variables at play. Establishing an association between two variables does not establish a causal relationship. It merely defines an observed relationship between the two variables. The regression coefficients describe the isolated association between any variable and the target variable using 3 pieces of information: the sign (direction of association), magnitude (size of association), and whether or not the association is statistically significant (is it unlikely we would observe the data if there were no association). This powerful statistical tool lets us make inferences about the relationships between variables in a population by analyzing a properly drawn sample. Similar to hypothesis testing, the same constraints and pitfalls surrounding inference apply. The regression coefficients computed for a sample distribute normally around the true association in the population according to a standard error, and if the sample is not representative, the regression analysis will yield results that do not generalize to the population.
Chapter 12: Problems with Regression
Regression analysis is a powerful tool when used correctly. Proper use requires an understanding that regression gives us clues, not truths, about associations between variables, and it should be used in conjunction with theoretical explanations to draw conclusions about data. When devising a regression equation, one must “estimate the equation”, which means you do not merely dump a plethora of variables into the equation, but you craft a theoretical reason for why you should add each explanatory variable. There are several common mistakes made when employing regression analysis that one should actively avoid: 1) using linear regression to analyze nonlinear relationships; 2) concluding causation from an association; 3) your dependent variable causes the explanatory variable; 4) omitting crucial variables; 5) using highly correlated explanatory variables; 6) extrapolating data to a different population; 7) adding too many variables to an equation.
Chapter 13: Program Evaluation
We know we cannot rely on correlations and assocations to determine causation, only that they give us clues into what to investigate. So how do we study cause and effect relationships? That is where program evaluation comes in, which is the statistical process of measuring the causal effect of an intervention. The key to program evaluation is to compare an intervention to its counterfactual, where the conditions are the same except there is no intervention. Ideally, researchers can construct the counterfactual as a control group in a randomized, controlled experiment. However, this is not always possible or ethical, in which cases the researchers have to be more clever and find naturally occurring conditions where the counterfactual can be approximated.
Conclusion
Statistics is a profound tool that can be wielded to do good in the world, but if used improperly, it can yield catastrophic consequences.