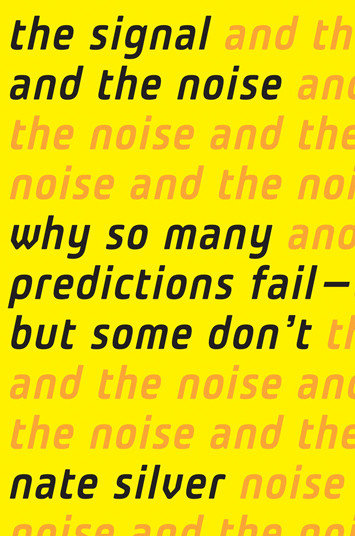
Summary and Review: The Signal and the Noise
Why So Many Predictions Fail - But Some Don't
✍️ The Author
This book was written by Nate Silver and published in 2012. Nate Silver is a statistician who founded FiveThirtyEight and was named one of Time’s 100 most influential people in 2009. He is well-known for correctly predicting 49 out of 50 states in the 2008 US Presidential election and all 50 states in the 2012 US Presidential election.
💡 Thesis of the Book
We live in times in which the available information at our fingertips is increasing exponentially, but most of that information is noise obscuring the signal. There are too many inputs for us to fully comprehend reality, so we have to simplify the inputs and construct approximations of reality. Many problems arise when we confuse what are approximations of reality with actual reality. Silver’s solution to navigate this complex landscape of information is to adopt Bayesian thinking. This means admitting to our biases and recognizing that we start from a position of subjective belief, and that we should expose ourselves to different representations of information to get a complete picture of the available evidence so that we can update our beliefs in the right direction.
💭 My Thoughts
Silver does an excellent job explaining the perils of information overload, the ease by which we mistake noise for signal, and best practices for navigating the complex landscape of information to find the signal buried in all the noise. As a Bayesian myself, I agree with the thesis of the book, which is that we should consider all hypotheses, think probabilistically about them, and update our beliefs using the Bayesian process. The book is also a gentle introduction to principles of forecasting, as Silver is an excellent forecaster himself and was able to convey some of the insights into succeeding at such an endeavor. My main takeaway from the book is that context turns information into knowledge. In order for our predictions to be useful, we have to understand the context of those predictions: uncertainty, unknown unknowns, and prior belief. To combat overconfidence and false positives, we should acknowledge the uncertainty in the systems we attempt to make predictions on, recognize the existence of information and possibilities we are completely unaware of, and utilize our prior beliefs via the Bayesian process.
📕 Chapter Summaries
Introduction
Humans are prediction machines. We evolved to be pattern-finding creatures, that is our biological strength. However, the explosion of information brought on first by the printing press, and now by the internet, has led to greater scientific, economic, and technological progress, but has triggered unintended higher order consequences regarding what we believe. The volume of information has outpaced our ability to process it and the noise-to-signal ratio is increasing. We are suffering from “information overload,” and it shows in our poor track record of predictions. We should never ground our decisions and beliefs in a naïve trust of model predictions. If a model predicts an outcome is impossible, that does not mean that outcome is impossible. Some of the most catastrophic prediction failures in history are a result of naïve trust in statistical instruments and algorithms. Every model and statistical instrument is based on a set of assumptions devised by humans, and history has shown our assumptions to be flawed. This does not mean we should toss data-driven predictions, but we should be cautious of what those predictions tell us.
A Catastrophic Failure of Prediction
The 2008-2009 financial crisis resulted from a catastrophic failure in several predictions made by several different entities. Homeowners and investors failed to predict that rising housing prices would lead to a housing bubble. Ratings agencies and banks failed to predict the risk of mortgage-backed securities. Economists failed to predict that the collapse of a highly-leveraged housing market would trigger a global financial crisis. Policymakers failed to predict the long-lasting damage to the economy that the financial crisis would inflict. All of these failures stem from the same mistake: considering historical data for an out-of-sample problem. The explosion of information has cursed us with a false sense of confidence in algorithmic prediction. We naïvely assume that an increase in available information correlates with an increase in our understanding of the world, when the opposite case is just as plausible. The 2008 financial crisis is just one recent example of how we have become overconfident in precise calculations and ignorant of the gap between our understanding and the truth. It is of vital importance that we humble ourselves, cautiously forecast, and recognize the prevalence of noise and the scarcity of signal.
Are You Smarter Than a Television Pundit?
Pundits and corporate media have a poor track record of making predictions, but interestingly enough, so do political scientists and analysts, those who get paid for accuracy. Research shows political forecasters don’t perform well when they let their ideologies influence their predictions, that is, they view the world through a narrow ideological lens and are incapable of examining complexity from multiple perspectives. They have trouble discerning between what they want the outcome to be from the outcome that is actually most probable. It is imperative that forecasters strive for objectivity, which does not merely mean being quantitative. Every “data-driven” forecast involves human decisions and assumptions, thus opening the door for human bias. Silver offers a few key principles to strive for objectivity: think probabilistically; do not be afraid to change your forecast given new information; look for consensus in information (use a variety of data sources); avoid reductionist models that employ a few “magic bullet” variables to predict complex phenomena; do not get lost in the narratives that manifest themselves in qualitative information; acknowledge to yourself that being “data-driven” is not synonymous with being “objective” (humans can influence models to fit their subjective beliefs).
All I Care About is W’s and L’s
The world of sports is a fun yet challenging way to flex your forecasting muscles. When it comes to sports, you want to account for the context in which statistics are gathered, separate skill from luck, and understand how a player’s performance changes as they age. When formulating the forecasting problem, the goal is to pinpoint the root factors. As the data becomes more high-level, meaning the number of influential factors and the role of chance is greater, you introduce more noise into your information sources, making it more difficult to find the signal. You want to think about the root factors, like athletic ability, that give rise to the higher-level statistics, like wins-losses. However, not all of these factors can be quantified. The reliance on numbers alone can leave gaps in your forecasting approach. Human judgement can help fill in these gaps by accounting for information that models do not. That is why hybrid approaches to sports forecasting can do very well. Sports teams rely on both statisticians and scouts, not one or the other, for a reason. The hybrid approach will yield more predictve power if the presence of human bias does not outweigh the benefit of incorporating additional qualitative information.
For Years You’ve Been Telling Us That Rain Is Green
Weather forecasting is often the butt of jokes, but it is actually a tale of success in the forecasting world. The weather is a very difficult system to predict because it both dynamic (current behavior influences future behavior) and nonlinear (variable relationships are not linear), and any system that is both dynamic and nonlinear is subject to chaos theory, which posits that predicting the future behavior of nonlinear, dynamic systems is tantamount to forecasting chaos because slight errors compound over time to produce dramatically different results. Our ability to predict the weather has improved significantly over the past several decades with the advent of compute power, better data collection, and greater domain knowledge, but our weather forecasting ability is still very constrained because of chaos theory. There are weather forecasting entities and weather reporting channels that report forecasts, but emphasis on accuracy is distorted among them. Some weather forecasters let other factors like political, economic, and social factors influence the forecast as opposed to striving for the best accuracy, whereas other forecasters seek to make and communicate the best forecast possible. The job of every forecaster, no matter the field, is to make the best forecast possible. To let external factors influence that process is a betrayal to the fundamental role of a forecaster.
Desperately Seeking Signal
The forecasting world features a plethora of forecasters that get caught in the trap of mistaking noise for signal because they are desperately searching for signal in a noisy world. Many forecasters don’t want to admit a particular system is just too noisy to make decent forecasts on, and they become obssessed with trying to find the signal, the pattern that explains it all. Earthquake forecasting is a great example of this. Scientists and mathematicians have been trying to predict earthquakes for centuries, but our ability to make those predictions has not progressed in the slightest, even with the advent of Big Data and greater computational power. Many forecasters have claimed to find the signal in earthquake prediction, but in every instance, future failed predictions debunks their claim and reveals they had only captured noise in the historical data. This is a problem known as overfitting, where a forecaster models noise in historical data rather than signal. The inverse problem is underfitting, where the model fails to capture all the signal, but overfitting is much more common. The prevalence of false discoveries can partly be explained by overfitting, but also by the prevalence of coincidence in a world of many predictions. It is unlikely that a prediction will come true by mere chance, but if the number of predictions made is high enough, it is actually quite likely that some of those predictions will be right by mere coincidence. Someone is bound to get lucky, and when they do, they will make headlines in the media, but eventually time will debunk their predictive methods. As Aristotle once said, “It is probable that improbable things will happen. Granted this, once might argue that what is improbable is probable.”
How to Drown in Three Feet of Water
Similar to the weather, the economy is a complex dynamic system that is challenging to predict. The difference lies in the theoretical basis, progress curves, and gravitation towards bias. While there has been tremendous improvement in weather forecasting, economic forecasting has experienced a flat progress curve: the forecasts have simply been terrible and show no signs of improving. This “failure” story can be explained by several factors. Economics still lacks a strong theoretical basis underpinning its dynamics and variable relationships. There are so many variables, bidirectional relationships, and feedback loops in an economic system, there is no fine line between independent and dependent variables. There are also millions of economic indicators, so there are a plethora of cases mistaking correlation for causation. Adding further complexity is the effect of policies on economic data. Historic economic data has to be contextualized by the policies in place at the time, and economists need to predict future policies in addition to their effects on the economy. The data economists have to work with is also incredibly noisy, as it is quite difficult to measure simple variables like GDP, and many studies have shown real-time estimates to be dramatically off. With plenty of error in the information sources, economists are already starting off on unstable grounds before they even get to the forecasting stage, which introduces a new issue. Economic forecasters are much more likely to succumb to personal bias and sway the forecasts in directions favorable to their political beliefs. With all of these effects at play on top of the tendency for economists to report forecasts without uncertainty intervals, economic forecasts are just not reliable and should be taken with a high dose of skepticism. While there are many economic forecasts that come true, it is almost always by mere chance. Somebody is going to get lucky. The key is to look at the consistency of a forecaster.
Role Models
The space of modeling infectious disease often runs into issues involving self-fulfilling predictions and self-cancelling predictions. A self-fulfilling prediction is one where the prediction of an outcome biases the system to producing that outcome, and so the predictor creates a false notion that it is truly predictive. A self-cancelling prediction is one where the prediction of an outcome biases the system to deviate away from that outcome, and so the prediction undermines itself. Infectious disease is a tough thing to model, especially in the early parts of an outbreak where the data is poor in quality and few in number, forcing epidemiologists to make faulty extrapolations. There has been success in “agent-based modeling”, which is a simulation approach. The success has not been in the ability to predict outbreaks, but in the ability to gain insight into how policies and broad behavior could effect the spread and inflicted harm of a disease. These models are not replacements of the universe. They are merely approximations, but some approximations can actually be very insightful. As the statistician George Box said, “All models are wrong, but some models are useful.”
Less and Less and Less Wrong
In the 18th century, an entire statistical philosophy emerged out of the simple idea of a minister named Thomas Bayes. His idea was that learning is a process of updating our prior beliefs about the world given new evidence, and that this process nudges our beliefs closer to the truth over time. The ideas of Bayes were formulated into a simple mathematical rule by mathematician Pierre-Simon Laplace known as Bayes theorem. This simple equation is the foundation of the branch of statistics called Bayesian statistics. The 20th century witnessed a divergence from Bayesian thinking as an intellectual countermovement gained steam. R.A. Fisher and his frequentist statistical philosophy challenged the Bayesian viewpoint of prior belief, calling it too subjective and a departure from objective science. The underlying idea behind frequentism is that all uncertainty stems from experiment design. Frequentist statistical methods ignore the bias of the researcher, whereas Bayesian statistical methods embrace such bias. The Bayesians are more skeptical of statistically significant results that are nonsensical, whereas frequentists only care about statistical significance since their prior beliefs are irrelevant. The dominant adoption of frequentist statistical significance tests by scientists and academic researchers has led to blurred lines between noise and signal, where now we really are unsure how many published experiments are actually true versus noise in the data. The criticism of frequentist statistics is starting to become more accepted and there is some growing resistance to these methods in the scientific and academic communities. Silver argues in favor of the Bayesian approach, “It will take some time for textbooks and traditions to change. But Bayes’s theorem holds that we will converge toward the better aproach. Bayes’s theorem predicts that the Bayesians will win.”
Rage Against the Machines
It was long thought that chess was the ultimate test of intelligence, and that machines could not beat the best chess players in the world because they lacked the creativity to play strategically. This belief was grounded in a flawed view of the game of chess. Chess is a game with no uncertainty. The state of the board is known at every given moment, and all boundaries and constraints are known to each player. However, a human or a machine could not possibly understand how any given move will play out toward the end because the search space is just too large to traverse in the limited amount of time. This is where prediction comes in. Players, and machines, need to make predictions about how certain moves will accomplish short-term goals given the current board configuration. Thus, the meta-objective of chess is to make better predictions than your opponent. Since predictions involve a series of calculations, and the chess board can be easily encoded, the game of chess actually plays into the primary advantage of computers over humans: computers are good at performing many calculations fast. That is why you will never see a human beat the best computer chess programs again.
The Poker Bubble
Poker is a game that combines luck and skill. The cards dealt are a random process, thus injecting luck into the game, but the ability to infer the opponent’s cards and make decisions based on the evidence (your cards, cards on the table, opponent’s decisions) is a demonstration of skill. A crucial aspect to this skill is the ability to predict the cards your opponents hold. Framed this way, to be a good poker player, you have to be a good Bayesian thinker. You have some prior belief about what the opponent’s are holding based on what they do after initially getting dealt their hand. As more cards are revealed on the table, you update this prior belief based on new evidence (what the opponent does in subsequent rounds) to arrive at a posterior belief of the range of cards that the opponents are likely to hold. Based on these predictions, you compare them with your own cards throughout the game to make your decisions. Silver introduces an idea he calls the Pareto Principle of Prediction, which is that 80% of the accuracy of a prediction comes from 20% of the effort, and this principle applies to poker. If you are playing with players that have got the basics of poker prediction down (most important 20%), then the gains of any additional skill are marginal, and the noise introduced from the randomness of the card dealing makes it difficult to consistently win. In fact, Silver suggests that if you want to make money off of predictions, you have to go into an area where there are plenty of “fish”, people who don’t even have the basics (crucial 20%) down. As you make bets on predictions, you have to recognize that the presence of randomness is going to make the results noisy, and that you cannot get fixated on the results. Rather than being results-oriented, you need to be process-oriented. Think about whether the decision-making process will lead to positive results on average as it is repeated again and again.
If You Can’t Beat ‘Em…
The stock market is a prime example of a Bayesian process, where the price represents the posterior belief about a company’s future earnings, undergoing continuous updates as new evidence emerges. This is the underlying idea behind Fama’s famous “efficient market hypothesis”, which posits that the market efficiently prices company stocks and nobody will be able to beat it consistently (make better predictions). This hypothesis has been the subject of major debate in economics and finance for decades. Many academics agree that investors cannot beat the market in the long run, but they tend to disagree that the market always efficiently prices the stocks. Sometimes, irrational behavior can dominate the market through a mechanism known as herding, where traders will mimic the behaviors of other traders, thus amplifying noise. The short-term perturbations in stock prices are a consequence of irrational trading behavior, which add noise to the signal. Many investors/traders have attempted to pinpoint statistical patterns in this noise or use fundamental analysis to predict short-term stock movements, but these efforts have not led to consistent above-market returns. Any additional earnings from statistical patterns are canceled out by the costs associated with executing trades more frequently and the inevitable disappearance of the pattern as more people discover it.
A Climate of Healthy Skepticism
Bayesian thinking and scientific progress go hand-in-hand. Our confidence in hypotheses should be grounded in underlying theory, and the data we collect should update the strengths of those beliefs. Just as it is easy to mistake noise for signal, it is also possible to mistake signal for noise. The signal can often be obscured by noise in the data, introduced by other factors and error in data collection. We can use scientific theory and our understanding of causal relationships to establish prior beliefs, making it more difficult for noisy data to convince us otherwise. In climate science, the greenhouse effect is a well understood theory, but can be difficult to notice in shorter time intervals because global temperature data is noisy. However, if you look at the broader trend over many decades, the signal becomes more clear: global temperatures are rising. There is a scientific consensus that anthropogenic climate change is a real phenomenon currently underway, but there is also a consensus that climate forecasts are not predictive of the future effects of climate change. This inaccuracy largely stems from the presence of various uncertainties, both near-term and long-term, as well as the lack of feedback that climate forecasters receive (climate forecasts span decades). There is plenty of skepticism of climate science, some of which is healthy and some of which is malign. The skepticism of the greenhouse effect is misplaced and nonsensical, but the skepticism of climate forecasts is healthy and warranted. We don’t really have a solid understanding of how the greenhouse effect will change aspects of the climate outside of temperatures and sea levels. Just because the climate is an incredibly complex system that is difficult to forecast does not mean we should give up. It just means we should adopt a Bayesian mindset, think about outcomes probabilistically, and admit to the uncertainties.
What You Don’t Know Can Hurt You
There are things we know, things we are aware that we don’t know, and then things that we don’t know we don’t know. The last part hits at the idea of “unknown unknowns”, which captures the fact that there are gaps in our knowledge that we are unaware of. We tend to only consider an incomplete set of events that could plausibly occur, and of that set there are events that we are unfamiliar with, and we tend to assign low likelihoods to those events because we mistake what is unfamiliar with what is improbable. All of these flaws in our thinking lead to improper preparation for events that are plausible to occur but live beyond the set of events we consider may happen. This is precisely how 9/11 occurred, which was an event that we did not consider beforehand but mathematics told us was plausible. If you look at the frequencies of terrorist attacks grouped by total fatalaties, you’ll find that terrorism data follows a power law distribution, similar to earthquakes. While terrorism attacks of magnitude are very infrequent, they are still expected to happen eventually according to some predicted time interval, and a 9/11-magnitude event was predicted to occur within several decades. Yet we did not think a hijacked plane incident would occur because the magnitude of such an event eclipses everything we have seen historically. When it comes to forecasting, it is important to keep in mind that you probably are not considering all the events, and that you should expand your horizon, keep an open mind, and think probabilistically about everything.
Conclusion
We live in times in which the available information at our fingertips is increasing exponentially, but most of that information is noise obscuring the signal. There are too many inputs for us to fully comprehend reality, so we have to simplify the inputs and construct approximations of reality. When we communicate information to each other, there is additional simplification to translate information into a linguistic structure. Many problems arise when we confuse what are approximations of reality with actual reality. Silver’s solution to navigate this jagged landscape of information is to adopt Bayesian thinking. This means admitting to our biases and recognizing that we start from a position of subjective belief, and that we should expose ourselves to different representations of information to get a complete picture of the available evidence so that we can update our beliefs in the right direction. Knowledge is information placed in context. Without context, information is dry and can be misleading. The context to prediction is uncertainty and prior belief, so when we fail to acknowledge this uncertainty and the presence of our prior assumptions, we can become overconfident in our predictions and mistake noise for signal. Don’t be a hedgehog. Strive for the truth. Strive for the signal. Avoid the noise, which are the distractions from the truth.