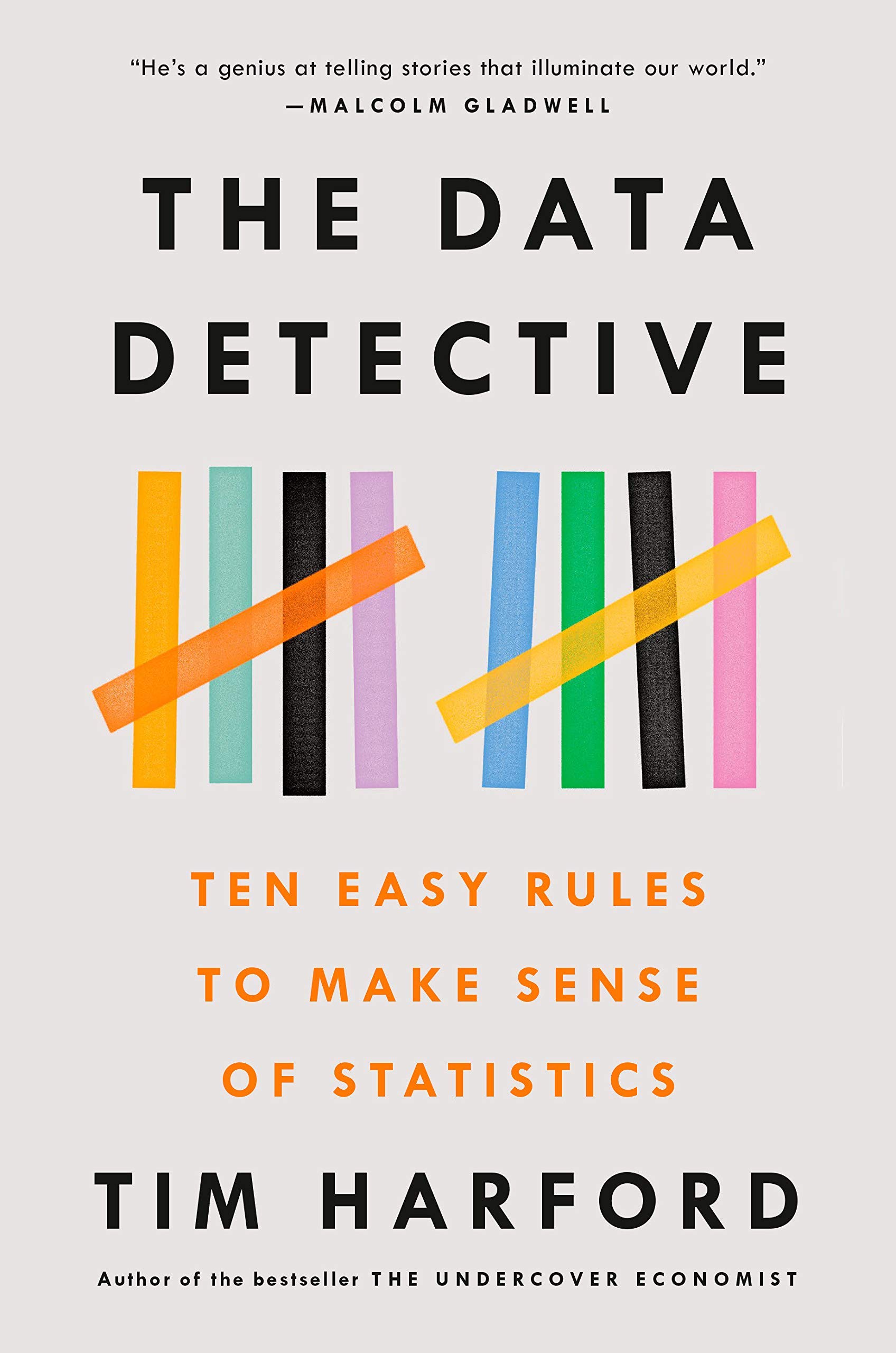
Summary and Review: The Data Detective
Ten Easy Rules to Make Sense of Statistics
✍️ The Author
This book was written by Tim Harford and published in 2021. Harford is an Oxford-based economist and BBC broadcaster who also writes for the Financial Times column, “The Undercover Economist.”
💡 Thesis of the Book
This book offers “ten commandments” of statistics, but if you distill all of them into one golden rule, it’s to be curious. By being curious about data, statistical methodology, and claims, you naturally arrive at the ten rules offered by this book. When presented with a statistical claim, the rules advise readers to consider:
- How they feel about the claim
- If the claim agrees with your personal experience
- If you understand what the claim actually means
- Information that puts the claim into context
- The backstory of the claim
- Who is missing from the data used to construct the claim
- Potential biases in the data used to produce the claim
- If the statistics underlying the claim originate from a government statistical agency or private company
- The persuasive, and potentially misleading, tactics of visualizations accompanying the claim
- That your initial thoughts about the claim could be wrong
💭 My Thoughts
As a quantitative person, I know it is easy to get lost in numbers, losing sight of the subtle human elements that regulate how we interpret numbers. The Data Detective helped me appreciate the importance of emotion, experience, deception, and psychology in the world of statistics. The commandments purveyed by this book makes it a statistical bible of sorts, one that I will keep in my backpocket and repeatedly reference in the future. I recommend anyone who interfaces with statistics read this book, digest the rules, and keep them close. The wisdom of the ten rules is sure to help anyone navigate the treacherous waters of noisy information and keep their emotions in check. While the thesis of the book is to be curious about data, my main takeaway was more specific, and that is the world is ruled by both feelings and numbers. Trying to escape that reality is a fool’s errand. To engage in sense-making, we must listen to both. Believing your views are emotionless and purely rational is a trap, and more dangerous than acknowledging the inescapable influence of your feelings. Feelings can be subtle, and if you ignore them, they can pull the strings without you realizing it. What you thought was rational was really whatever made you feel good. You must confront the numbers and your feelings about them.
📕 Chapter Summaries
Introduction: How To Lie With Statistics
One of the most popular books in the field of statistics is Darrell Huff’s “How To Lie With Statistics”. This written work takes a cynical view on statistics, that it is a trickster’s tool for fooling people into believing falsehoods. Harford takes the opposite approach with this book, which offers a more optimistic take on the discipline. We must acknowledge that statistics can be used to mislead us in the wrong direction, but more importantly, it is crucial to leading us in the right direction. Rather than giving up on evidence and believing whatever makes us feel good, we ought to engage in truth-seeking because it helps us see the world more clearly. It may seem daunting to navigate the complex landscape of data, but with the right frame of mind, you can do it.
Rule 1: Search Your Feelings
There are a lot of steps between analyzing evidence and reaching a conclusion, and if you do not control your emotions, your feelings about the particular matter will manipulate the steps you take to confirm your preconceptions. This pyschological phenomenon is known as “motivated reasoning”, and the solution to avoiding this bias is not to be more informed or intelligent. In fact, people with greater expertise and intelligence are more susceptible to motivated reasoning because they have more mental tricks and information to creatively lay out a path connecting the data to the conclusion that satisfies their feelings. The feelings set the destination, and the smarts find a way to get you there. Additionally, expertise can give a false sense of confidence that you know what you are talking about. You don’t doubt your conclusion because you are an “expert”, but little do you know it wasn’t the “expert” in you that reached the conlusion. Feelings can, and often do, override expertise. If you don’t confront your feelings and recognize the enormous bias it can throw in the thinking process, being smart and knowledgable is, ironically, a weakness. So pay attention to how you emotionally react to data or statistical claims. Search your feelings.
Rule 2: Ponder Your Personal Experience
What should we do when our personal experience contradicts the statistics? This is a tricky question, because it depends on the circumstances. Sometimes both are true. Personal experience is a worm’s eye-view on reality, where we see a local subset of broader reality in detail, and statistics is a bird’s eye-view, giving insight into the big picture. Both can be useful, but avoid conflating personal experience with an approximation of universal reality. This is known as naïve realism, and it’s naïve because the assumption is that the information we collect via personal experience is not biased in any way, that it is a representative sample of the global truth. For truth-seeking, it is best to use our personal experience as a check on statistics, and if there is contradiction, investigate why. Consider the possibility your individual perspective is too narrow and does not capture the broader situation like the statistics do, but also consider the possibility the statistics may not report what you think they do. Typically, it is best to err on the side of statistics because it can aggregate experiences on much larger scales than you can, but use personal experience as a way of investigating what those statistics actually look like on the ground level. Ponder your personal experience.
Rule 3: Avoid Premature Enumeration
Problems in statistics often stem from words, not numbers. You can start a statistical analysis on faulty ground if you fail to precisely define what is being measured, a mistake Harford calls “premature enumeration”. Statistical tools will crunch the numbers regardless of what terminology you assign to the numbers, but how humans interpret the results is a function of both the number crunching and the words associated with those numbers. Whenever you come across statistical results, always look for precise definitions of what they are counting, even if it does not seem ambiguous. We all have a basic idea of what inequality represents, but definitions of inequality in the context of an economic study have a profound effect on the results. Partisan academics and institutions take advantage of the ambiguity in measure definition by searching for a definition that produces the conclusion they want. Clarify what the data truly represents, and it becomes more difficult to be fooled by published statistical results or your own statistical analyses due to a misalignment between what you think the data captures and what it actually captures. Avoid premature enumeration.
Rule 4: Step Back and Enjoy the View
We tend to view the world through a narrow temporal perspective. We think about what is happening right now, and we forget that there is context to our current situation and the data we see. Various news outlets operate on different rhythms, where some are fast-paced and cover stories on a daily or hourly timeline, while others are more broadly focused with a coverage timeline of months or years. However, the media is dominated by fast-paced news reporting, and in order to compete for attention, they only report what is sudden and surprising. As Steven Pinker has observed, good things tend to happen gradually over time, and bad things happen suddenly. This tendency skews the high frequency news towards negative stories, since most surprising stories are negative, and the plethora of slowly developing good stories go unpublished. Why is it humans don’t feel worried about their local situation, but are stressed over what is happening at a more societal level? This is expected, since our information on society at large is passed to us through a negatively biased news filter. Don’t fall into this narrow view on statistics. Whenever you come across data or claims, broader the time horizon of that data, look at the context, get a sense of scale, compare it to other situations, and often you will get a better understanding of what is actually going on. Step back and enjoy the view.
Rule 5: Get the Backstory
The key point of this rule is to understand the backstory of the claim. Survivorship bias is sneaky and can make false claims seem quite reasonable from a statistical standpoint. If data has been dropped from a sample by nonrandom means, and you are only presented the data that “survives”, the data paints a nonrepresentative picture of the population that it originated from. By examining the backstory, you can incorporate the data that did not survive to form a complete view of the data and reach proper conclusions. Publication bias is an increasingly alarming form of survivorship bias that manifests itself in the research paper publishing process, where scientists are starting to realize that positive results are more likely to be published than negative results, thus distorting the landscape of published supporting evidence. When presented with positive findings, ask how many experiments were conducted. Are there published or unpublished negative findings? One experiment is not enough to fully support a statistical claim. Get the backstory.
Rule 6: Ask Who Is Missing
One of the most stealthiest statistical mistakes lurking in the closet is ommission. Data can have gaps in it, and if we feed that data to an algorithm, the results will not account for the missing data. Sampling bias describes systematic errors in drawing observations from a population that results in an unrepresentative sample. This means the sample has ommitted relevant subsets of the data that will skew any downstream analysis. Sampling bias is much more dangerous and subtle than sampling error, which is the inherent error in random sampling. This is why efforts are better spent on ensuring proper sampling rather than increasing the raw size of the sample. So whenever you are presented with a statistical claim, or are doing statistical analysis of your own, consider any possible ommissions in the underlying data that may skew the results. We cannot assume we would reach the same conclusion if the ommitted data were included. Ask who is missing.
Chapter 7: Demand Transparency When the Computer Says No
In the 21st century, there has been massive hype behind Big Data and learning algorithms which led many to question the future need for sampling methods, theoretical models, and even the scientific method. Then, a wave of hysteria surrounding Big Data followed, purveying claims that algorithms are not trustworthy and mining large “found datasets” for patterns does not actually work. Harford argues that we should be skeptical of both the hype and hysteria. He references the ideas of philosopher Onora O’Neil, who argues that trust should be discriminatory along two dimensions: what we are trusting and what we trust it do. Rather than ask if we can trust algorithms, we should ask which algorithms can we trust and for which tasks. The best ways to build trust in specific algorithms for specific scenarios is to allow for transparency into its predictions, prove its effectiveness in a randomized control trial, and demonstrate better predictive power than its human counterparts. In some cases, Big Data does hold useful patterns that humans are unaware of, and in other cases, Big Data is biased and “garbage”. Large datasets are susceptible to the same problems as small datasets. If the data is of high quality, the learning algorithms shine. If the data is garbage, the learning algorithms fail miserably. This is why we need transparency. If we don’t know why an algorithm makes a certain prediction, we can’t determine if it is driven by a truth or a bias captured in the data collection process. Demand transparency when the computer says no.
Rule 8: Don’t Take Statistical Bedrock for Granted
Statistical agencies serving government form the statistical bedrock of a nation. While it is possible they become corrupted or coerced into doing the bidding of politicians, the most politically neutral statistical institutions have been those of Western civilizations. These agencies have gathered crucial statistics that inform policymaking, producing a massive ROI over time (how can you make good policy decisions without knowing anything about your country?). In a world of missing data and deceiving statistics, we should be grateful for the reliable statistics that are published by these agencies, as they allow for a stable structure for statisticians to work on. Don’t take statistical bedrock for granted.
Rule 9: Remember that Misinformation can be Beautiful, Too
Visualizations of data can be beautiful, but don’t let the dazzle of a graphic add credibility to the data it presents. It is just as easy to craft a beautiful visualization of low quality data as it is for high quality data. Additionally, visualizations can be more than mere informative tools, they can be persuasive tools. The way you visualize data can influence how someone digests that data and reaches a conclusion. Whenever you are presented with a nice visual presentation of data, don’t let the aesthetics fool you. Think about how it makes you feel and what conclusions you instinctively draw. This may be what the designer wants you to feel and conclude. In visual form, misinformation thrives just as much as truth, perhaps even more so. Always be sure to dig deeper than the surface and analyze the elements of the visualization to determine if this is an honest representation of data or a trojan horse for faulty conclusions. Remember that misinformation can be beautiful, too.
Rule 10: Keep an Open Mind
A crucial quality for engaging in any kind of sense-making is open mindedness. If you are to understand the world more clearly through statistics or any other kind of means, you must be willing to change your perspectives, approaches, and conclusions. How likely are you to be right the first time? The truth is not likely. Researcher Phillip Tetlock has investigated what makes a good forecaster, and he found the ability to keep an open mind is key to forecasting success. “For superforecasters, beliefs are hypotheses to be tested, not treasures to be guarded,” Tetlock says. We should all think about our beliefs in this way, as hypotheses. The scientific process is all about challenging hypotheses, not finding roundabout ways to justify them despite evidence to the contrary. Science is a tried and true way of understand the world more clearly, and it would be wise to model our own sense-making process after it. Numbers are an informative way to view the world, but how we feel about them can distort that view. We tend to make mistakes not in the raw quantitative analysis, but when we refuse to accept what the data tells us or what it cannot tell us. Keep an open mind.
Golden Rule: Be Curious
This book offers “ten commandments” of statistics, but if you distill all of them into one golden rule, it’s to be curious. By being curious about data, statistical methodology, and claims, you naturally arrive at the ten rules offered by this book. While greater scientific literacy leads to increased polarization and motivated reasoning, studies show greater scientific curiosity leads to the opposite. Neuroscientists have shown that incurious people react to challenges to their beliefs as they do to life-threatening situations. Opposing views cause anxiety for those who lack curiosity, but for the curious ones, they find differing views interesting and intriguing, as an opportunity to explore new ideas and opinions. So how do we become more curious? Loewenstein’s “information gap” theory of curiosity posits that gaps between what we know and want to know generates curiosity, and curiosity fuels unbiased learning. If we think we know everything, this gap shrinks to zero, and if we know nothing, we don’t even know what could be known. The trick is to know enough to know what we don’t know and to maintain humility as we acquire more knowledge. Do this, and you will be in the right frame of mind for understanding the world through data. Be curious.